This article was generated with the assistance of artificial intelligence. However, all content has been thoroughly reviewed and curated by a human editor before posting to guarantee accuracy, relevance, and quality.
As forensic DNA profiling continues to advance, the sheer volume of data generated by technologies like capillary electrophoresis (CE) and massively parallel sequencing (MPS) has become increasingly complex to analyze. Enter machine learning (ML), an innovative computational approach that has already transformed fields such as finance, medicine, and autonomous vehicles. Now, forensic scientists are beginning to explore its potential in forensic DNA analysis. In a critical review published in Forensic Science International: Genetics, Mark Barash and his team provide an in-depth examination of how ML algorithms can streamline forensic DNA profiling, improve accuracy, and reduce human error. From short tandem repeat (STR) genotyping to DNA mixture interpretation, the study outlines the current applications of machine learning in forensic DNA and highlights the challenges that remain.
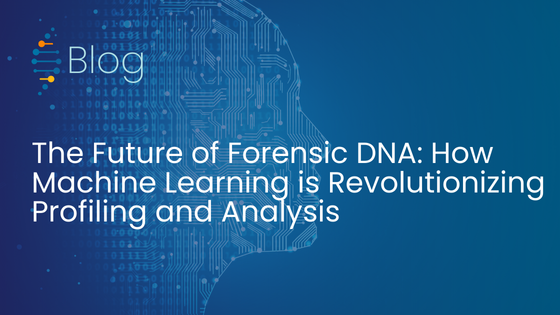
Machine Learning in Forensic DNA: A Promising Collaboration
Machine learning refers to a set of algorithms that can learn from data without explicit programming. In forensic DNA analysis, where the data are often vast and complex, ML can be used to find patterns, make predictions, and assist in decision-making. While traditional methods have relied on human expertise for data interpretation, ML offers a way to process large datasets more efficiently and identify subtle correlations that may be missed through manual analysis.
The potential for ML in forensic science is clear, but it is still an emerging field within the forensic community. One of the reasons for this slow adoption is the gap in understanding between forensic scientists and machine learning experts. Many forensic scientists are unaware of the capabilities of ML, while data scientists may not fully appreciate the unique requirements of forensic analysis. Nonetheless, the authors of the review argue that with proper validation, ML could play a critical role in improving the speed and reliability of DNA profiling.
Applications of Machine Learning in STR Genotyping
One of the key areas where ML is being applied in forensic DNA analysis is in the interpretation of STR data. STR profiling remains the gold standard for forensic DNA analysis, but interpreting the results—particularly in mixed samples—can be challenging. Existing tools like GeneMapper™ assist with allele calling, but they require manual confirmation, which is time-consuming and prone to errors.
Machine learning models can take STR genotyping to the next level by automating the process of allele designation. Instead of relying on static analytical thresholds (where peaks below a certain height are disregarded), ML models use dynamic thresholds or even no thresholds at all. This enables the model to learn from raw electropherogram (EPG) data, taking into account the entire dataset and making predictions that maximize the information extracted from DNA evidence.
Several studies have demonstrated the potential of ML for STR allele designation. For example, the review highlights work by Adelman and colleagues, who developed a symbolic regression model that automatically detects and removes pull-up peaks from EPG data. This approach achieved a predictive accuracy of 96% when used with dynamic thresholds, outperforming traditional static threshold methods.
The benefits of ML extend beyond accuracy. By automating routine tasks such as artefact detection and allele calling, machine learning can significantly reduce the time required to analyze DNA samples, freeing up forensic analysts to focus on higher-level tasks. Additionally, models that incorporate artificial neural networks (ANNs) have shown promise in eliminating the need for manual electropherogram interpretation altogether.
Massively Parallel Sequencing and the Role of ML
Massively parallel sequencing (MPS) offers forensic scientists the ability to analyze more comprehensive genetic information than traditional CE methods. However, the increase in data volume and complexity also introduces new challenges. MPS provides detailed information on DNA sequences, including the STR loci and their flanking regions, but this abundance of data requires sophisticated bioinformatic tools for accurate analysis.
Here again, machine learning proves valuable. In their review, Barash and colleagues discuss how ML tools can help with the extraction of STR sequences from MPS data. The study highlights Fragsifier, a software that uses a random forest model to detect STR loci by analyzing sequences of repeating nucleotides. This tool significantly outperforms traditional methods by utilizing both the repeat sequences and their flanking regions, offering a more complete analysis of the data.
The challenges posed by MPS data—such as sequencing errors and artefacts—are well-suited for machine learning’s strengths in pattern recognition. As more forensic labs transition to MPS for forensic applications, the demand for machine learning solutions will only increase. The ability of ML to handle massive datasets, detect errors, and provide accurate predictions makes it a critical tool in the future of forensic DNA analysis.
Enhancing DNA Mixture Interpretation with Machine Learning
One of the most challenging aspects of forensic DNA analysis is the interpretation of DNA mixtures. As the number of contributors to a DNA sample increases, so does the complexity of accurately identifying individuals within the mixture. In cases with degraded DNA or low-template samples, distinguishing true alleles from noise and artefacts becomes even more difficult.
Current probabilistic genotyping systems (PGS) assist forensic analysts by calculating likelihood ratios that quantify the probability of a DNA profile under two competing hypotheses. However, these systems rely on manual inputs, such as the estimated number of contributors (NoC) to a mixture, which can introduce human bias and errors.
Machine learning offers a solution by automating this process. The review discusses several ML models developed to estimate the NoC in DNA mixtures, including NOCIt, a continuous probabilistic model that incorporates peak heights, drop-in, drop-out, and other variables to predict the number of contributors. According to Barash and colleagues, NOCIt demonstrated superior accuracy compared to traditional methods when tested on a large set of DNA profiles.
Another promising tool, PACE™, uses support vector machine (SVM) algorithms to classify DNA samples and predict the number of contributors with greater than 90% accuracy. The model also automates the identification of artefacts such as stutters and pull-ups, significantly reducing the potential for human error.
Despite these advancements, machine learning models still face limitations in the interpretation of complex DNA mixtures. The review notes that while ML models like NOCIt and PACE™ show great promise, they require extensive validation before they can be widely implemented in forensic casework. Variability in training datasets and system parameters remains a challenge, and more standardized datasets are needed to ensure the robustness and reproducibility of these models.
Challenges and Limitations of Machine Learning in Forensic DNA
While machine learning has clear potential to revolutionize forensic DNA analysis, there are several limitations that must be addressed before it can become a routine tool in forensic labs. One of the biggest challenges is the “black box” nature of many ML algorithms. Unlike traditional methods, where each step in the analysis is transparent, ML models can be difficult to interpret, raising concerns about their use in legal contexts where transparency is critical.
The review calls for the adoption of explainable AI (XAI) techniques, such as decision trees and rule-based models, to make machine learning more transparent and interpretable. These techniques can help forensic analysts understand how the model arrived at a particular result, increasing confidence in the predictions made by the algorithm.
Additionally, machine learning models require extensive training datasets to perform accurately. For forensic DNA analysis, this means collecting and labeling vast amounts of genetic data, which can be both time-consuming and expensive. Furthermore, forensic labs may lack the computational resources needed to run these models efficiently, further hindering their widespread adoption.
Challenges and Limitations of Machine Learning in Forensic DNA
As forensic DNA analysis continues to grow in complexity, machine learning offers a powerful solution for streamlining workflows, improving accuracy, and reducing human error. From STR genotyping to DNA mixture interpretation, the potential applications of ML in forensic science are vast. However, as with any new technology, these tools must be thoroughly validated and made more transparent before they can become standard practice in forensic labs.
The future of forensic DNA analysis lies in the integration of machine learning, but to fully realize its potential, collaboration between forensic scientists and data scientists will be essential. As more forensic labs embrace this technology, machine learning is poised to become an indispensable tool in the quest for more accurate, reliable, and efficient forensic investigations.
Citations
Barash, M., McNevin, D., Fedorenko, V., Giverts, P. (2024). Machine learning applications in forensic DNA profiling: A critical review. Forensic Science International: Genetics, 69, 102994. https://doi.org/10.1016/j.fsigen.2023.102994